Four Key Lessons for ML Model Security & Management
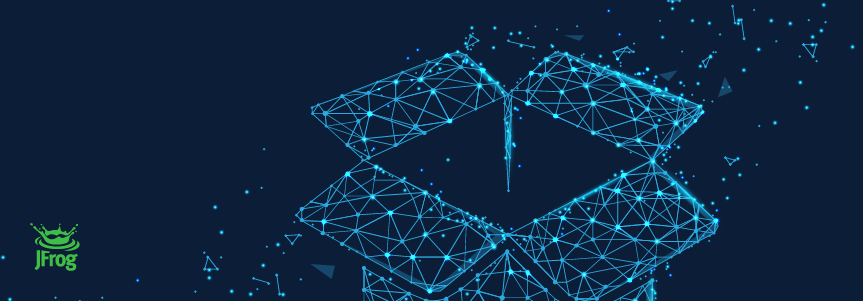
With Gartner estimating that over 90% of newly created business software applications will contain ML models or services by 2027, it is evident that the open source ML revolution is well underway. By adopting the right MLOps processes and leveraging the lessons learned from the DevOps revolution, organizations can navigate the open source and proprietary ML landscape with confidence. Platforms like JFrog, that include ML model management capabilities can further support organizations in their journey towards successful adoption.
Since the first open source package from the GNU Project was released by Richard Stallman in 1983, there has been a huge evolution of software reproducibility, reliability, and robustness. Concepts such as Software Development Life Cycle Management (SDLC), Software Supply Chain (SSC), and Release Lifecycle Management (RLM) have become a cornerstone of how to manage and secure software development environments.
In terms of MLOps, here are four lessons covering topics specifically related to AI Models including:
- Traceable versioning schemas
- Artifact caching and availability
- Model and dataset licensing
- Finding trusted open source ML repositories
As enterprises increasingly embrace machine learning models and services, it becomes crucial to leverage open source packages while simultaneously ensuring security and compliance. Open source models and datasets offer numerous benefits, but they also come with their own set of challenges and risks. Here we will explore some key lessons learned from the DevOps revolution and see how they can be applied to ensure successful adoption of open source ML models.
Lesson 1 – Adopt a clear, traceable versioning schema
Versioning allows an organization to be sure the software they create is using the right parts. With good versioning you can rollback bad deployments and necessitate fewer patches for live customers experiencing bugs in the application.
In the traditional world of software development, Semantic Versioning (SemVer) is the standard. Semantic Versioning is a very powerful tool, but can only reflect a single timeline. With Semantic Versioning you can identify the present and past, as well as the order between them.
When it comes to ML Model Versioning, however, the case is considerably different. While software builds with the same inputs should be consistent, with ML models two sequential training sessions can lead to totally different results. In ML model training, versioning schemas have many dimensions. Training might be done in parallel, using different parameters or data, but in the end all training results require validation. Your versioning schema should consist of enough metadata so that both Data Scientist, DevOps, ML Engineers and SRE will find it easy to understand the version’s content. While many ML tools use some form of Semantic Versioning, JFrog is taking a different approach to ML model versioning that better accommodates the complexity of ML model development and multiple stakeholders involved in the process.
In the traditional world of software development, Semantic Versioning (SemVer) is the standard. Semantic Versioning is a very powerful tool, but can only reflect a single timeline. With Semantic Versioning you can identify the present and past, as well as the order between them.
When it comes to ML Model Versioning, however, the case is considerably different. While software builds with the same inputs should be consistent, with ML models two sequential training sessions can lead to totally different results. In ML model training, versioning schemas have many dimensions. Training might be done in parallel, using different parameters or data, but in the end all training results require validation. Your versioning schema should consist of enough metadata so that both Data Scientist, DevOps, ML Engineers and SRE will find it easy to understand the version’s content. While many ML tools use some form of Semantic Versioning, JFrog is taking a different approach to ML model versioning that better accommodates the complexity of ML model development and multiple stakeholders involved in the process.
Lesson 2 – Cache every artifact you use as they might disappear
Not all open source projects can be relied upon for the long term. Some might close down, while in other cases companies may stop supporting packages they created, meaning that the latest version might not work as well as the previous one.
To protect against this type of instability, when working with ML models, it is advised to cache everything that you use as part of training or inference. This includes: The model, the software packages, the container you run it in, the data, parameters, features, and more. Even the ML model itself is a piece of software, so it is wise to cache all those packages as well. There are various caching tools in the market, including JFrog Artifactory with ML model support, covering the most popular ML package types.
To protect against this type of instability, when working with ML models, it is advised to cache everything that you use as part of training or inference. This includes: The model, the software packages, the container you run it in, the data, parameters, features, and more. Even the ML model itself is a piece of software, so it is wise to cache all those packages as well. There are various caching tools in the market, including JFrog Artifactory with ML model support, covering the most popular ML package types.
Lesson 3 – Model and dataset licensing procedures
Open source does not mean free! Most open source models have a license agreement that states what you can and can not do. Licensing is a very complex field and you might want to consult with a legal expert before selecting a model with a license that might put your company’s assets at risk. There are tools in the market to enforce licensing compliance, such as JFrog Curation and JFrog Xray that ensure your software licenses are compliant with company policy.
Lesson 4 – Use open source ML models from trusted sources only
When integrating open source into your software, you are de-facto putting your trust in the software creator to maintain the quality, security, and maintenance levels you need to ensure your software runs smoothly. Unfortunately, it is quite common to use an open source package, only to find out later on that there is a critical bug and the maintainer is not capable of solving it. As a last resort, you can use your own development resources to get into the code and start patching it – after all it is open source software – but in reality it is easier said than done and even worse, requires resources for maintaining the code going forwards.
Enterprises need to come up with a set of rules that determine if an open source package or model is mature enough to be used by their developers. JFrog’s best practice recommendations advise to at least look at the number of contributors and the date last release, as well other relevant information. The JFrog Platform can assist in this effort by automating policies to make your developers’ life easier and more productive.
Enterprises need to come up with a set of rules that determine if an open source package or model is mature enough to be used by their developers. JFrog’s best practice recommendations advise to at least look at the number of contributors and the date last release, as well other relevant information. The JFrog Platform can assist in this effort by automating policies to make your developers’ life easier and more productive.
Jump into the open source ML revolution with confidence
When it comes to ML models, versioning becomes more complex due to the multiple dimensions involved in training and validation. Caching every artifact used, becomes essential to mitigate the risks associated with the instability of open source projects.
It is also crucial to consider the quality, security, and maintenance levels provided by the software creator, taking into consideration critical bugs that may be detected down the line, requiring companies to allocate their own resources for maintenance.
By adopting lessons learned from the DevOps revolution and applying them to the open source ML landscape, MLOps professionals can better navigate the challenges and harness the benefits of ML models effectively, securely and efficiently.
Adopting the right MLOps processes today will set you up for success tomorrow. Check out JFrog’s ML model management capabilities and key industry partnerships, to see for yourself how they can support and improve your ML development operations by scheduling a demo or starting a free trial.
It is also crucial to consider the quality, security, and maintenance levels provided by the software creator, taking into consideration critical bugs that may be detected down the line, requiring companies to allocate their own resources for maintenance.
By adopting lessons learned from the DevOps revolution and applying them to the open source ML landscape, MLOps professionals can better navigate the challenges and harness the benefits of ML models effectively, securely and efficiently.
Adopting the right MLOps processes today will set you up for success tomorrow. Check out JFrog’s ML model management capabilities and key industry partnerships, to see for yourself how they can support and improve your ML development operations by scheduling a demo or starting a free trial.